七个鲜为人知的搜索网站
Pandas being the most widely used data analysis and manipulation library provides numerous functions and methods to work with data. Some of them are used more frequently than others because of the tasks they perform.
熊猫是使用最广泛的数据分析和处理库,它提供了许多处理数据的功能和方法。 由于它们执行的任务,它们中的一些比其他使用更频繁。
In this post, we will cover 4 pandas operations that are less frequently used but still very functional.
在本文中,我们将介绍4种不常用的熊猫操作,但它们仍然非常有用。
Let’s start with importing NumPy and Pandas.
让我们从导入NumPy和Pandas开始。
import numpy as np
import pandas as pd
1.分解 (1. Factorize)
It provides a simple way to encode categorical variables which is a required task in most machine learning techniques.
它提供了一种编码分类变量的简单方法,这是大多数机器学习技术中必需的任务。
Here is a categorical variable from a customer churn dataset.
这是来自客户流失数据集的分类变量。
df = pd.read_csv('/content/Churn_Modelling.csv')df['Geography'].value_counts()
France 5014
Germany 2509
Spain 2477
Name: Geography, dtype: int64
We can encode the categories (i.e. convert to numbers) with just one line of code.
我们可以只用一行代码对类别进行编码(即转换为数字)。
df['Geography'], unique_values = pd.factorize(df['Geography'])
The factorize function returns the converted values along with an index of categories.
factorize函数返回转换后的值以及类别索引。
df['Geography'].value_counts()
0 5014
2 2509
1 2477
Name: Geography, dtype: int64unique_values
Index(['France', 'Spain', 'Germany'], dtype='object')
If there are missing values in the original data, you can specify a value to be used for them. The default value is -1.
如果原始数据中缺少值,则可以指定要用于它们的值。 默认值为-1。
A = ['a','b','a','c','b', np.nan]
A, unique_values = pd.factorize(A)
array([ 0, 1, 0, 2, 1, -1])A = ['a','b','a','c','b', np.nan]
A, unique_values = pd.factorize(A, na_sentinel=99)
array([ 0, 1, 0, 2, 1, 99])
2.分类 (2. Categorical)
It can be used to create a categorical variable.
它可用于创建分类变量。
A = pd.Categorical(['a','c','b','a','c'])
The categories attribute is used to access the categories:
Categories属性用于访问类别:
A.categories
Index(['a', 'b', 'c'], dtype='object')
We can only assign new values from one of the existing categories. Otherwise, we will get a value error.
我们只能从现有类别之一分配新值。 否则,我们将获得值错误。
A[0] = 'd'

We can also specify the data type using the dtype parameter. The default is the CategoricalDtype which is actually the best one use because of memory consumption.
我们还可以使用dtype参数指定数据类型。 默认值为CategoricalDtype,实际上这是最好的一种用法,因为它会消耗内存。
Let’s do an example to compare memory usage.
让我们做一个比较内存使用情况的例子。
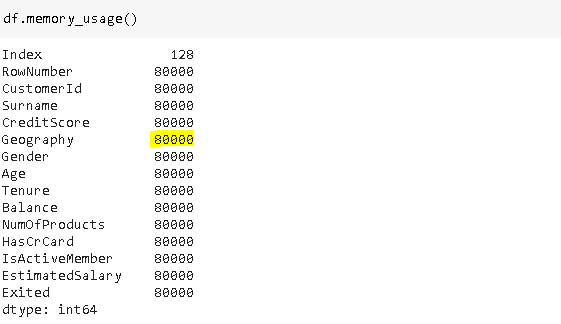
This is the memory usage in bytes for each column.
这是每列的内存使用量(以字节为单位)。
countries = pd.Categorical(df['Geography'])
df['Geography'] = countries
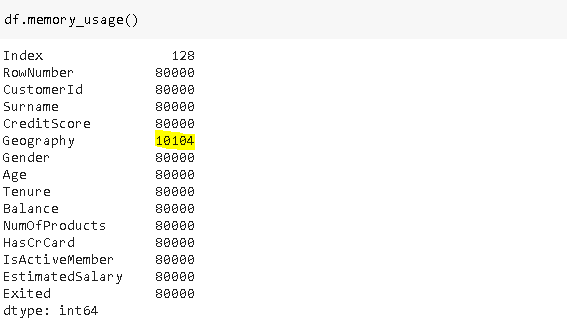
The memory usage is 8 times less than the original feature. The amount of memory saved will further increase on larger datasets especially when we have very few categories.
内存使用量比原始功能少8倍。 在较大的数据集上,保存的内存量将进一步增加,尤其是在类别很少的情况下。
3.间隔 (3. Interval)
It returns an immutable object representing an interval.
它返回一个代表间隔的不可变对象。
iv = pd.Interval(left=1, right=5, closed='both')3 in iv
True5 in iv
True
The closed parameter indicates if the bounds are included. The values it takes are “both”, “left”, “right”, and “neither”. The default value is “right”.
close参数指示是否包括边界。 它采用的值是“ both”,“ left”,“ right”和“ noth”。 默认值为“ right”。
iv = pd.Interval(left=1, right=5, closed='neither')5 in iv
False
The interval comes in handy when we are working with date-time data. We can easily check if the dates are in a specified interval.
当我们使用日期时间数据时,该间隔会很方便。 我们可以轻松地检查日期是否在指定的间隔内。
date_iv = pd.Interval(left = pd.Timestamp('2019-10-02'),
right = pd.Timestamp('2019-11-08'))date = pd.Timestamp('2019-10-10')date in date_iv
True
4.宽到长 (4. Wide_to_long)
Melt converts wide dataframes to long ones. This task can also be done with the melt function. Wide_to_long offers a less flexible but more user-friendly way.
Melt将宽数据帧转换为长数据帧。 该任务也可以通过熔化功能来完成。 Wide_to_long提供了一种不太灵活但更加用户友好的方式。
Consider the following sample dataframe.
考虑以下示例数据帧。
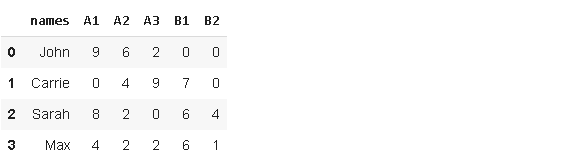
It contains different scores for some people. We want to modify (or reshape) this dataframe in a way that the score types are represented in a row (not as a separate column). For instance, there are 3 score types under A (A1, A2, A3). After we convert the dataframe, there will only be on column (A) and types (1,2,3) will be represented with row values.
它对某些人包含不同的分数。 我们希望以分数类型在一行中(而不是在单独的列中)表示的方式修改(或重塑)此数据框。 例如,A下有3种得分类型(A1,A2,A3)。 转换数据框后,将仅在(A)列上,并且类型(1,2,3)将用行值表示。
pd.wide_to_long(df, stubnames=['A','B'], i='names', j='score_type')
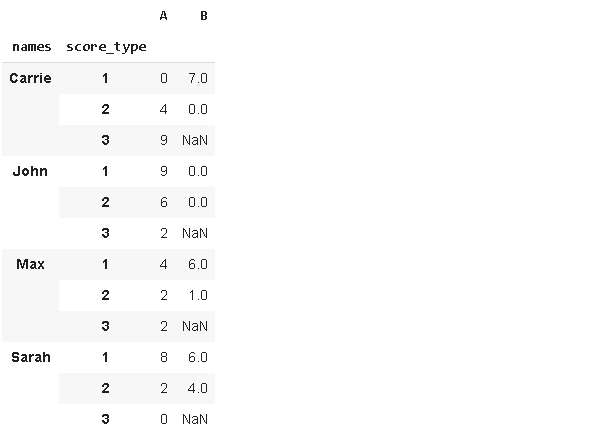
The stubnames parameter indicates the names of the new columns that will contain the values. The column names in the wide-format need to start with the stubnames. The “i” parameter is the column to be used as the id variable and the ‘j’ parameter is the name of the column that contains subcategories.
stubnames参数指示将包含值的新列的名称。 宽格式的列名称必须以存根名称开头。 “ i”参数是用作id变量的列,“ j”参数是包含子类别的列的名称。
The returned dataframe has a multi-level index but we can convert it to a normal index by applying the reset_index function.
返回的数据帧具有多级索引,但是我们可以通过应用reset_index函数将其转换为普通索引。
pd.wide_to_long(df, stubnames=['A','B'], i='names', j='score_type').reset_index()
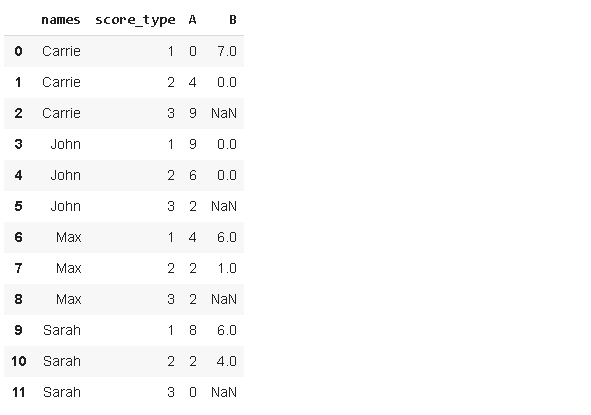
Pandas owes its success and predominance in the field of data science and machine learning to the variety and flexibility of the functions and methods. Some methods perform basic tasks whereas there are also detailed and more specific ones.
熊猫公司在数据科学和机器学习领域的成功和优势归功于功能和方法的多样性和灵活性。 一些方法执行基本任务,但也有详细且更具体的方法。
There are usually multiple ways to do a task with Pandas which makes it easily fit specific tasks well.
通常,有多种方法可以对Pandas执行任务,这使其很容易适应特定任务。
Thank you for reading. Please let me know if you have any feedback.
感谢您的阅读。 如果您有任何反馈意见,请告诉我。
翻译自: https://towardsdatascience.com/4-less-known-yet-very-functional-pandas-operations-46dcf2bd9688
七个鲜为人知的搜索网站
本文来自互联网用户投稿,该文观点仅代表作者本人,不代表本站立场。本站仅提供信息存储空间服务,不拥有所有权,不承担相关法律责任。如若转载,请注明出处:http://www.luyixian.cn/news_show_747437.aspx
如若内容造成侵权/违法违规/事实不符,请联系dt猫网进行投诉反馈email:809451989@qq.com,一经查实,立即删除!